How my computer is like an old coach's brain (only better!)
Alan Couzens, M.Sc. (Sports Science)
Nov 7th, 2017
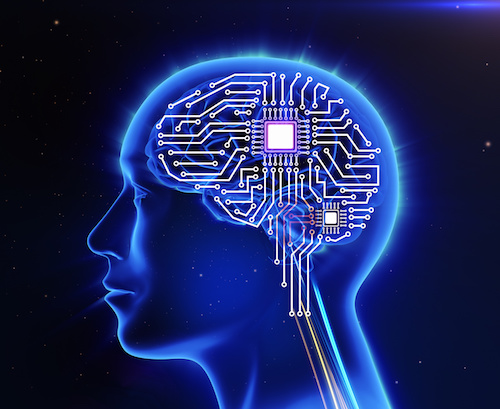
I had an interesting twitter conversation yesterday after posting this link to a review paper on the importance of quantifying training load. Matt Dixon replied with the following…
Y. (to add for clarity) = the key for any coach is to not dogmatically fall into ONLY holding onto gut OR only data to assess/make decisions
— Matt Dixon (@purplepatch) November 6, 2017
These sorts of tweets praising the ‘gut feeling’ of coaches over a data-driven approach (or even putting them on the same level), when it comes to guiding decisions, always get a bit of a rise out of me. Probably because…
a) The term 'using data to assess/make decisions' needs to be better defined. How are you using the data? I question just how much data science is truly taking place in current coaching (i.e. the distinction having a Training Peaks account & collecting data from an athlete's gadgets vs actually pulling that data down & using it to develop/refine models that guide rational, actionable decisions). In my (admittedly based) view, if you’re going to talk about the shortcomings of ‘the data approach’, it demands some background knowledge/experience in data science and…
b) The term ‘gut feeling’ also need to be better defined. Frankly, I don't really know what that is! What does 'following your gut' mean? Is it following your whim? Is it silencing thoughts & rolling with the first instinct that comes to the fore? As someone who has been called a ‘robot’ on more than one occasion :-), I’m not sure I get it. I can't say I’ve ever had a ‘gut feeling’ or at least, I’m not sure I’ve ever labelled it as such.
Personally, I think that the first step in continuing to learn and progress as coaches is to be able to clearly identify and define what we currently base our programming decisions on. To say that I base my decisions on "gut feel" is, to me, a bit of a cop-out & this lack of clear definition does nothing to further our cause of learning from one another & progressing our field. So, in order to assess the true merit of these ‘gut feelings’ to the efficacy of coaching, I think it’s important to at least start by trying to define the term.
So, what do we mean when we say ‘gut feeling’?
The best definition I can come up with is…
A visceral recognition of some undefinable pattern that lies deep within our long term memory.
i.e. while we may not be able to explicitly recall all of the specific experiences that led to its development, our current experience triggers some kind of deeply hidden memory in our limbic system of a pattern that we have experienced in the past.
More specific to our domain, maybe we see an athlete who is failing Seiler’s ‘hair in the yogurt test’ at the breakfast table and our brain harkens back to other times that we saw the same thing in similar athletes who went on to a painful (emotionally registering) season-ending bout of overtraining. So our ‘gut’ says, “ooooh I don’t want to experience that again so let’s ease up on training for a bit.”
These kinds of simple assessments happen in training squads around the globe, where a coach ‘eyeballs’ a particular athlete and classifies them accordingly like so…
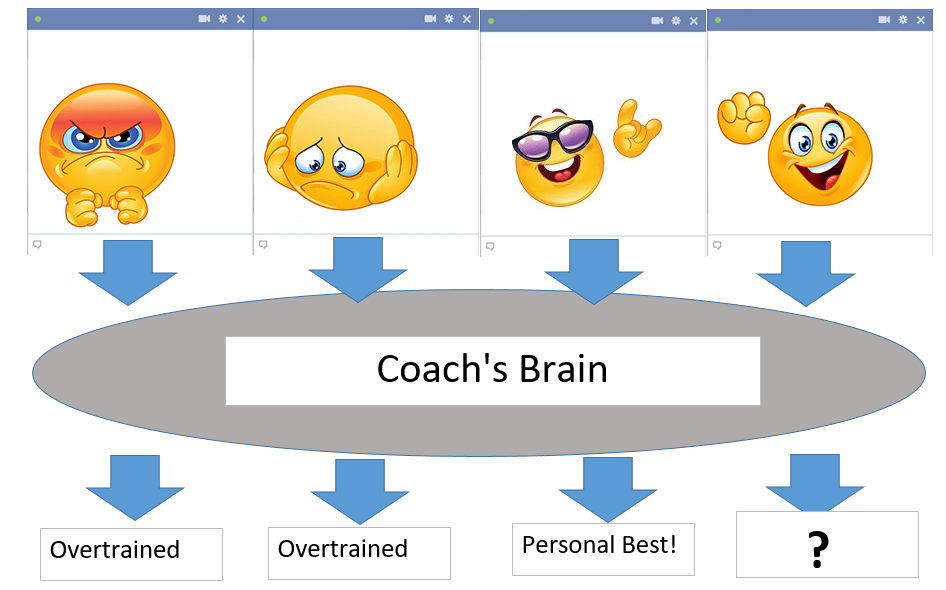
If you had to guess what lies in the question mark box under the face of the happy athlete, you could probably do a pretty good job simply by looking at the other faces and seeing which one he most resembles
In the simplified example above, the coach learns – grumpy athlete often precedes overtraining, sad athlete often precedes overtraining, chillaxed happy athlete often precedes PR and so when he/she sees a similar expression in a new athlete they might predict training is in a good spot for a strong performance.
In computer terms, the above process of predicting new patterns on the basis of previously observed patterns is called supervised machine learning – the algorithm (brain) is ‘trained’ on previous – input->output data, then new data is presented to the brain and the brain is asked to predict what the outcome will be.
By this definition, 'gut feelings' and 'data models' may not be all that different after all. In fact, we might say that they lie on the same continuum, with the indefinable 'black box' pattern links of a computer neural network being very similar to the same indefinable 'black box' pattern links of an old coach's 'gut' but they differ in one important way - the amount of information behind the predictive power, i.e they're essentially the same process only one isn't anywhere near as predictively strong as the other. Let me explain...
Almost without exception, in the computer world, the more training data that the brain/algorithm factors in when making its prediction, the better those predictions are.
The above speaks to the importance of experience in coaching. The more data we are exposed to, the more refined these patterns in the brain should become and the better our predictions should be. At least in theory…
The reason that predictive ability doesn't scale with experience in 'real life' is that it assumes that the Coach can hold all of this data that he/she is exposed to over the years in our heads & draw on it at will. I don’t know about you, but as a coach who is rapidly approaching ‘old coach’ status (if not already there), while my experience keeps growing, my data storage faculties don’t! I’m not embarrassed to admit that, after dealing with literally hundreds of athletes over my past 20+ years of coaching, my ability to recall the particulars of a given season for a given athlete is not what it used to be. In fact, sometimes, I will find myself looking over my database trying to recall a face after seeing a name of an athlete that I worked with many years ago.
Maybe my experience is that of early senility but I’ve a hunch that, while it’s not often admitted, it’s more like the norm. The fact of the matter is, as human beings, our CPU processing power far exceeds the memory storage capacities of our RAMs and our inability to pull and query large amounts of previous data greatly limits the power of the models that we are able to come up with. Wouldn’t it be great if we had an external ‘hard drive’ that we could plug into so we have direct access to all of the experience that we’ve accumulated over the years? You mean, like a computer? :-)
By employing a machine to take some of the load off our brain and act as a second 'experience receptacle' we are able to make decisions from a position of far more context. And, more to the point, by quantifying this information, we are able to make decisions from a position of statistical context. Going back to our example above of 'looking an athlete in the face' to determine if they are too tired, what does a 'significantly' tired face look like? I know some athletes who look perpetually hagged. It's just the way their face is :-) Then I know others who always look gleeful and like the energizer bunny even in the midst of fatigue. Clearly we need the individual baseline provided by long term data to make sense of this one off assessment. Additionally, I *want* to see my athletes somewhat tired through most of the training year. They have to experience tiredness to get better! So what does *too* tired look like? Where does the threshold lie? Is it 4 'crows feet' lines instead of 3? These kinds of assessments clearly have limitations & they require the Coach to keep a running 'crows feet' tally for each athlete in their head at all times :-)
In a more practical sense, if we ask an athlete to rate their tiredness on a 1-5 scale, some athletes will be, how should I say, more 'sensitive' to tiredness than others. Other athletes will be like the fighting Knight from Monty Python - even on death's door, "It's just a flesh wound" let's keep fighting! By quantifying it, we are able to establish a baseline mean for each athlete. Athlete a's 'norm' might be a tiredness of 4, while athlete b's might be a tiredness of 2. For each we're then able to assess any worthwhile change from their norm. The real value of the "how are you feeling?" question lies not so much in their answer to the question but in how their answer compares to their own personal norm.
Yes, by plugging in a 'second brain' we have access to much more data that we can draw on when identifying patterns and formulating hypotheses. By maintaining access to all of this data that we accumulate over time & using it to continuously refine and improve our models in a methodical, systematic, mathematic way, we are able to keep and harness the full predictive power that comes with all of this real life data, all of this real world experience.
In addition to the ability to store a Terabyte or more of data from your life experiences, a further benefit to employing a machine to help with our data analysis is that a computer doesn't sift data through the same filters of belief and bias that we humans do....
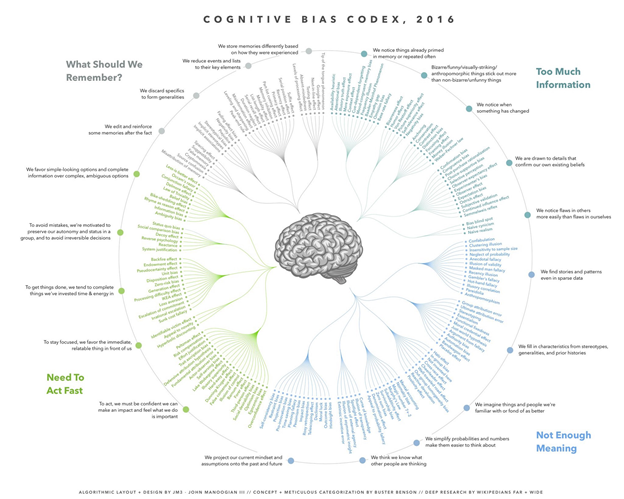
With the limitations in processing power mentioned above, our brain develops ‘shortcuts’ - processor-saving heuristics like the 'recency bias' that weights information that we have more immediate access to with higher importance than older memories. Additionally our brains are protective of us and tend to frame data in a light that is positive to us. Computers don’t do this. They have no problem amending patterns in the light of new evidence even if it contradicts what they previously may have said (or tweeted all over social media :-) they don’t see the penalty of an ego-hit in doing this, the only number they are interested in maximizing is the true accuracy of their predictions.
I have to concede, computers are still currently lacking in one area mentioned in the above exchange – eyeballing.
Computer vision is one of the toughest areas of artificial intelligence to crack but it is progressing at breakneck rates (as anyone with a Facebook page knows). Thanks to revolutionary new algorithms like Convolutional Neural Networks, we can now identify specific individuals from pictures, even when those pictures are taken in different contexts or from different angles. Beyond that, companies like Affectiva are now applying computer vision to detect specific emotions in people. It will not be long before this trickles down to using ‘selfies’ to identify training readiness of an athlete. The technology is already there for this, it is just a matter of developing a sufficiently large dataset to train the algorithm on & when we do, it will have better predictive accuracy than a coach because it will be able to retain thousands and thousands of previous images of a given athlete in its ‘brain’ at one time and will be able to generate predictions on all of this data. It will exceed human prediction because it will be scaled against the athlete’s personal baseline and it will be able to detect any worthwhile change in both directions – sympathetic and parasympathetic. As experienced coaches know, sometimes a perpetually hyped up, ‘raring to go’ athlete is even more of a red flag than an athlete who is obviously exhausted.
In short, when it comes to the pattern recognition of ‘gut feelings’, we humans have some serious limitations in both our memory capacity and our biases. While our intelligence as a species is unsurpassed, it is only through the recognition of (& making efforts to overcome) these limitations that we will be able to achieve our true potential in any field, including our humble domain of maximizing athletic performance.
Coach smart,
AC
TweetDon't miss a post! Sign up for my mailing list to get notified of all new content....